The Impact of High-Density Urban Wind Environments on the Distribution of COVID-19 Based on Machine Learning: A Case Study of Macau
Abstract: The COVID-19 epidemic poses a global challenge. Urban wind environments, as integral parts of cities, may contribute to virus spread. Understanding their impact is vital for effective prevention strategies. This study employs a conditional generative confrontation network (CGAN) to train a model using simulated urban wind and COVID-19 distribution data. The model predicts COVID-19 distribution probability under various wind environments, revealing their relationship.
Key findings: (1) Different wind environments show significant variations in COVID-19 distribution, with high building density areas being more susceptible. (2) COVID-19 hotspots in building complexes correlate with building characteristics. (3) Building area influences COVID-19 spread. Post-epidemic planning in high-density cities can consider building houses on the northeast side of mountains, adopting “strip” or “rectangular” layouts, and ensuring the long side faces southeast. (4) Overall wind speed around buildings should exceed 2.91 m/s, with an optimal range of 4.85-8.73 m/s. These findings inform urban planning and public health strategies for more effective prevention and control. This study utilizes machine learning to uncover the impact of urban wind environments on COVID-19 distribution, providing crucial insights for planning and public health. [SCITIP]
DOI: https://doi.org/10.3390/buildings13071711
CITE: Zheng, Liang, Yile Chen, Lina Yan, and Jianyi Zheng. 2023. “The Impact of High-Density Urban Wind Environments on the Distribution of COVID-19 Based on Machine Learning: A Case Study of Macau” Buildings 13, no. 7: 1711. https://doi.org/10.3390/buildings13071711
作者的话
1. 心得分享
今日分享之论文,是本月见刊上线的第一篇 SCIE 期刊论文,按照目前的学习计划安排,这一篇应该是这个月唯一的一篇的。
特别强调:这一篇论文有“Feature Paper”标识。如官方解释所示:
Feature papers represent the most advanced research with significant potential for high impact in the field. A Feature Paper should be a substantial original Article that involves several techniques or approaches, provides an outlook for future research directions and describes possible research applications.
Feature papers are submitted upon individual invitation or recommendation by the scientific editors and must receive positive feedback from the reviewers.
专题论文代表了最先进的研究,在该领域具有巨大影响力的巨大潜力。专题论文应该是一篇实质性的原创文章,涉及多种技术或方法,提供对未来研究方向的展望并描述可能的研究应用。专题论文是根据科学编辑的个人邀请或推荐提交的,并且必须得到审稿人的积极反馈。
上期预告也有提及,《The Impact of High-density Urban Wind Environments on the Distribution of COVID-19 Based on Machine Learning: A Case Study of Macau》(基于机器学习的高密度城市风环境对 COVID-19 分布的影响:以澳门为例)一文于本月在线发表在期刊 Buildings(ISSN: 2075-5309)(SCIE、Scopus)之 Special Issue “The Adaptability of Residential Planning and Design to World-Changing Events”(住宅规划和设计对世界变化事件的适应性)。本期重点介绍一下。
本人为共一作者,在本次论文中主要是从写作、全文翻译(含改写)、写 coverletter、返修改动了 1 轮、投稿、校稿 1 轮等。返修确实是一个很头大的事情,这一次活生生返修多了 5 -6p 的版面,我还要想审稿人这个疑问,系统回应了,但是对应的文字插入哪里,怎么把原来的逻辑顺下来。综合来看,我个人感受,返修比写第一稿更费时间。返修完了在第二轮那会,审稿人 2 给了看上去非常高的评价:“The paper has been revised correctly. I can recommend it to be published now, as it presents very high scientific level.”
![[JCR] Urban Wind Environments on the Distribution of COVID-19 [JCR] Urban Wind Environments on the Distribution of COVID-19](https://scitip.com/wp-content/uploads/2023/07/61b19338cce2863c3ab9486dfbea58e2.png)
中间很长一段时间是整个澳门特区的风环境的图卡住了,为了出图,中间辗转了很多。这个重大功劳要归功于我们家娜娜,最后跑了一张全澳门的风环境超级叼图。
原图可 152MB 呢老铁~!打晕我绑架我我都不会给的一张图。
![[JCR] Urban Wind Environments on the Distribution of COVID-19 [JCR] Urban Wind Environments on the Distribution of COVID-19](https://scitip.com/wp-content/uploads/2023/07/image.png)
2. 作者解读
![[JCR] Urban Wind Environments on the Distribution of COVID-19 [JCR] Urban Wind Environments on the Distribution of COVID-19](https://scitip.com/wp-content/uploads/2023/07/image-1-1024x594.png)
线上阅读地址:https://www.mdpi.com/2075-5309/13/7/1711
引用格式:
Zheng, Liang, Yile Chen, Lina Yan, and Jianyi Zheng. 2023. “The Impact of High-Density Urban Wind Environments on the Distribution of COVID-19 Based on Machine Learning: A Case Study of Macau” Buildings 13, no. 7: 1711. https://doi.org/10.3390/buildings13071711
大白话解释一下这篇论文:说来话长,这个征稿其实是去年就收到了,后面做了别的事情以后,在 DDL 第一生产力的促进之下,终于给投了出去。熟悉我的小伙伴都知道,一年前澳门是大爆发了 COVID-19 当时我也很久不出门,年底 + 今年年头发了两篇关于 COVID-19 和城市形态的论文,然后那 500 个病例的数据还在,刚好,延续了之前的研究,叠加了一个风环境的元素。然而,时过境迁,现在讲 COVID-19 显得有点过时,但我们更想强调,这是一种规划设计前期的方法,澳门这个 case 只是我们讲解这项方法的一个例题。understand?在本文中,探讨了以下六个问题:(参考正文的 1.3. Problem Statement and Objectives)
- 以中国澳门为例,高密度的城市空间及其塑造的城市风环境对 COVID-19 的分布有何影响?
- 机器学习技术如何协助分析 COVID-19 的分布?
- 进一步,根据 2022 年 6 月中国澳门 500 例 COVID-19 爆发的足迹数据,COVID-19 与城市风环境之间的相关性如何?
- 不同形态布局下城市风环境如何促进或抑制 COVID-19 的分布?
- 采用哪种形式的可持续居住区布局规划设计,更有利于适应流行病环境?
- 在后疫情时代,这项研究能为其他类似疫情提供哪些思考?
Keywords: machine learning; COVID-19; urban wind environment; high density city; urban planning; urban public health
Institution:Faculty of Humanities and Arts, Macau University of Science and Technology
Funding: This research was funded by the National Social Science Foundation’s special academic team project for unpopular research (21VJXT011).
论文标题框架:
1. Introduction
1.1. Research Background
1.2. Literature Review
1.2.1 The Pandemic and the Urban Wind Environment
1.2.2 Application Areas of Machine Learning in COVID-19
1.2.3 COVID-19 and Housing Conditions
1.3. Problem Statement and Objectives
2. Materials and Methods
2.1 Data collection
2.2 Data processing
2.3 CGAN method
3. Model Training
3.1 Model training process and verification
3.2 Correlation Analysis of the Wind Environment and COVID-19 in Different Building Layout Types
3.3 Robustness test of the model
4. Discussion: Residential Planning under Long COVID
4.1 Typical Residential Building Types in Low-Epidemic-Risk Areas
4.2 Wind environment simulation and epidemic situation analysis and verification
4.3 Design principles
5. Conclusions
Appendix A
Appendix B
References
文章插图
1. 研究背景
![[JCR] Urban Wind Environments on the Distribution of COVID-19 [JCR] Urban Wind Environments on the Distribution of COVID-19](https://scitip.com/wp-content/uploads/2023/07/image-2-1024x376.png)
![[JCR] Urban Wind Environments on the Distribution of COVID-19 [JCR] Urban Wind Environments on the Distribution of COVID-19](https://scitip.com/wp-content/uploads/2023/07/image-3-1024x317.png)
2. 材料准备
![[JCR] Urban Wind Environments on the Distribution of COVID-19 [JCR] Urban Wind Environments on the Distribution of COVID-19](https://scitip.com/wp-content/uploads/2023/07/image-4-1024x317.png)
![[JCR] Urban Wind Environments on the Distribution of COVID-19 [JCR] Urban Wind Environments on the Distribution of COVID-19](https://scitip.com/wp-content/uploads/2023/07/image-5-1024x334.png)
![[JCR] Urban Wind Environments on the Distribution of COVID-19 [JCR] Urban Wind Environments on the Distribution of COVID-19](https://scitip.com/wp-content/uploads/2023/07/image-6-1024x333.png)
3. 模型训练
![[JCR] Urban Wind Environments on the Distribution of COVID-19 [JCR] Urban Wind Environments on the Distribution of COVID-19](https://scitip.com/wp-content/uploads/2023/07/image-7-1024x378.png)
![[JCR] Urban Wind Environments on the Distribution of COVID-19 [JCR] Urban Wind Environments on the Distribution of COVID-19](https://scitip.com/wp-content/uploads/2023/07/image-8-854x1024.png)
![[JCR] Urban Wind Environments on the Distribution of COVID-19 [JCR] Urban Wind Environments on the Distribution of COVID-19](https://scitip.com/wp-content/uploads/2023/07/image-9-1024x368.png)
![[JCR] Urban Wind Environments on the Distribution of COVID-19 [JCR] Urban Wind Environments on the Distribution of COVID-19](https://scitip.com/wp-content/uploads/2023/07/image-10-1024x368.png)
4. 模型 测试
![[JCR] Urban Wind Environments on the Distribution of COVID-19 [JCR] Urban Wind Environments on the Distribution of COVID-19](https://scitip.com/wp-content/uploads/2023/07/image-11-1024x612.png)
![[JCR] Urban Wind Environments on the Distribution of COVID-19 [JCR] Urban Wind Environments on the Distribution of COVID-19](https://scitip.com/wp-content/uploads/2023/07/image-12-1024x612.png)
![[JCR] Urban Wind Environments on the Distribution of COVID-19 [JCR] Urban Wind Environments on the Distribution of COVID-19](https://scitip.com/wp-content/uploads/2023/07/image-13-925x1024.png)
![[JCR] Urban Wind Environments on the Distribution of COVID-19 [JCR] Urban Wind Environments on the Distribution of COVID-19](https://scitip.com/wp-content/uploads/2023/07/image-14-925x1024.png)
5. 统计、分析、归纳、总结
![[JCR] Urban Wind Environments on the Distribution of COVID-19 [JCR] Urban Wind Environments on the Distribution of COVID-19](https://scitip.com/wp-content/uploads/2023/07/image-15-1024x506.png)
![[JCR] Urban Wind Environments on the Distribution of COVID-19 [JCR] Urban Wind Environments on the Distribution of COVID-19](https://scitip.com/wp-content/uploads/2023/07/image-16-1024x536.png)
![[JCR] Urban Wind Environments on the Distribution of COVID-19 [JCR] Urban Wind Environments on the Distribution of COVID-19](https://scitip.com/wp-content/uploads/2023/07/image-17-1024x237.png)
![[JCR] Urban Wind Environments on the Distribution of COVID-19 [JCR] Urban Wind Environments on the Distribution of COVID-19](https://scitip.com/wp-content/uploads/2023/07/image-19-1024x235.png)
![[JCR] Urban Wind Environments on the Distribution of COVID-19 [JCR] Urban Wind Environments on the Distribution of COVID-19](https://scitip.com/wp-content/uploads/2023/07/image-18-1024x234.png)
![[JCR] Urban Wind Environments on the Distribution of COVID-19 [JCR] Urban Wind Environments on the Distribution of COVID-19](https://scitip.com/wp-content/uploads/2023/07/image-20-1024x238.png)
![[JCR] Urban Wind Environments on the Distribution of COVID-19 [JCR] Urban Wind Environments on the Distribution of COVID-19](https://scitip.com/wp-content/uploads/2023/07/image-21-1024x238.png)
![[JCR] Urban Wind Environments on the Distribution of COVID-19 [JCR] Urban Wind Environments on the Distribution of COVID-19](https://scitip.com/wp-content/uploads/2023/07/image-22-1024x238.png)
![[JCR] Urban Wind Environments on the Distribution of COVID-19 [JCR] Urban Wind Environments on the Distribution of COVID-19](https://scitip.com/wp-content/uploads/2023/07/image-23-1024x736.png)
![[JCR] Urban Wind Environments on the Distribution of COVID-19 [JCR] Urban Wind Environments on the Distribution of COVID-19](https://scitip.com/wp-content/uploads/2023/07/image-24-1024x530.png)
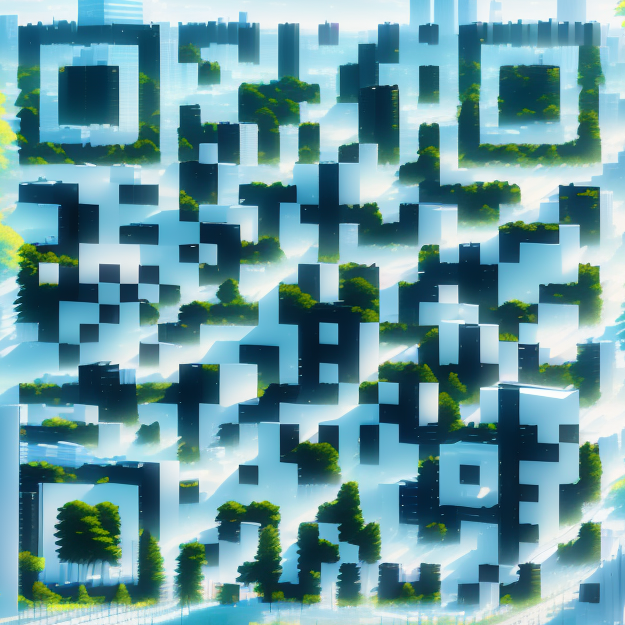