Nondestructive Testing Research on the Surface Damage Faced by the Shanhaiguan Great Wall Based on Machine Learning
Abstract: The Shanhaiguan Great Wall is a section of the Great Wall of the Ming Dynasty, which is a UNESCO World Heritage Site. Both sides of its basic structure are composed of rammed earth and gray bricks. The surface gray bricks sustain damage from environmental factors, resulting in a decline in their structural quality and even a threat to their safety. Traditional surface damage detection methods rely primarily on manual identification or manual identification following unmanned aerial vehicle (UAV) aerial photography, which is labor-intensive. This paper applies the YOLOv4 machine learning model to the gray surface bricks of the Plain Great Wall of Shanhaiguan as an illustration. By slicing and labeling the photos, creating a training set, and then training the model, the proposed approach automatically detects four types of damage (chalking, plants, ubiquinol, and cracking) on the surface of the Great Wall. This eliminates the need to expend costly human resources for manual identification following aerial photography, thereby accelerating the work. Through research, it is found that (1) compared with manual detection, this method can quickly and efficiently monitor a large number of wall samples in a short period of time and improve the efficiency of brick wall detection in ancient buildings. (2) Compared with previous approaches, the accuracy of the current method is improved. The identifiable types are increased to include chalking and ubiquinol, and the accuracy rate increases by 0.17% (from 85.70% before to 85.87% now). (3) This method can quickly identify the damaged parts of the wall without damaging the appearance of the historical building structure, enabling timely repair measures.[SCITIP]
DOI: https://doi.org/10.3389/feart.2023.1225585
CITE: Qian Li, Liang Zheng, Yile Chen, Lina Yan, Yuanfang Li and Jing Zhao. 2023. “Nondestructive Testing Research on the Surface Damage Faced by the Shanhaiguan Great Wall Based on Machine Learning” Frontiers in Earth Science. Volume 11 – 2023. doi: 10.3389/feart.2023.1225585
作者的话
1. 心得分享
今日分享之论文,是 8 月录用的第 2 篇 SCIE、Scopus 期刊论文,如上期预告所提及,《Non-destructive Testing Research on the Surface Damage Faced by the Shanhaiguan Great Wall Based on Machine Learning》(基于机器学习的山海关长城表面损伤之无损检测研究)一文于 9 月 4 日也就是今天在线发表在瑞士 Frontiers Media S.A. 出版的 SCIE、Scopus 期刊《Frontiers in Earth Science》之中,分类栏目为 Sec. Atmospheric Science。本篇推文来着重介绍一下。
本人为共同通讯作者,在本次论文中主要是写作、全文翻译(含改写)、返修改动了 1 轮、投稿等。中间也比较折腾吧,例如审稿人 1 没有按时提交审查报告,一直在延迟,例如处理的学术编辑找了一个月才有匹配的,例如返修那会失联了得兜底。返修的时候审稿人 2 希望我引用他的文章,然后副主编空降在互动论坛叫我不要引用他……最后就是替换了一些文献,论坛盖的楼很高,到了 7 楼给的是一个大修,一个小修,反正就是逐点回应,润了一下色,稿件提交了,审稿人再复审都同意了出版,次日就显示文章录用了。之前谁说 OA 很快给钱就能发,我这里折腾了 3 个月了都,还不如上一篇非 OA 的从投稿到返修到录用的 21 天。玄学,妙不可言。
![[JCR]Great Wall on Machine Learning [JCR]Great Wall on Machine Learning](https://scitip.com/wp-content/uploads/2023/09/image-1.png)
![[JCR]Great Wall on Machine Learning [JCR]Great Wall on Machine Learning](https://scitip.com/wp-content/uploads/2023/09/image-1024x494.png)
![[JCR]Great Wall on Machine Learning [JCR]Great Wall on Machine Learning](https://scitip.com/wp-content/uploads/2023/09/image-2-1024x732.png)
Production Forum 里面其实也有一些隐藏的信息,例如,顶上显示“Article scheduled to be published”,基本就是这几天没差了。同时也可以看这里面的文件有没有 EPUB 的压缩包,有的话其实可以下载,里面有一个 OPS 文件夹有 xhtml 格式,就能看到文章如何被安排得明明白白。
![[JCR]Great Wall on Machine Learning [JCR]Great Wall on Machine Learning](https://scitip.com/wp-content/uploads/2023/09/image-3-1024x432.png)
![[JCR]Great Wall on Machine Learning [JCR]Great Wall on Machine Learning](https://scitip.com/wp-content/uploads/2023/09/image-4-1024x471.png)
![[JCR]Great Wall on Machine Learning [JCR]Great Wall on Machine Learning](https://scitip.com/wp-content/uploads/2023/09/image-5-1024x804.png)
(所以这也是为什么我能确定是具体上线的日期,看这个截图底部的时间,8 月底已经知道了)
![[JCR]Great Wall on Machine Learning [JCR]Great Wall on Machine Learning](https://scitip.com/wp-content/uploads/2023/09/image-6-1024x551.png)
PO 一下时间线:
![[JCR]Great Wall on Machine Learning [JCR]Great Wall on Machine Learning](https://scitip.com/wp-content/uploads/2023/09/image-7.png)
还申请了个录用证书:(因为制作周期半个月到一个月不等,怕同学急用,有跟客服申请)
![[JCR]Great Wall on Machine Learning [JCR]Great Wall on Machine Learning](https://scitip.com/wp-content/uploads/2023/09/image-8.png)
2. 简介
大白话解释一下:通过机器学习中的 YOLOv4 的框架,构建一种对于无损检测图像分析的方法,case 是山海关长城。山海关长城作为明长城的一部分,是著名的世界遗产,其平原长城部分的基本结构是内部位为夯土两侧表面包青砖。在自然气候影响下,表面的灰砖会产生一些病害,导致其结构质量下降,甚至威胁到它们的安全。传统的表面病害检测主要是依据人工踏勘辨别或无人机航拍后人工辨别, 这将耗费巨大的人力资源。本论文使用 YOLOv4 机器学习模型,以山海关长城的表面青砖为例,通过对照片进行切片标注,创建训练集,然后进行模型训练,实现自动查找长城表面灰砖的四种病害(粉化、苔藓及植物、泛碱、断裂)类型,这将解决航拍后的人工辨别问题,使工作进展得更快。当然,同样都是青砖,与之前的澳门世界遗产缓冲区的青砖传统建筑也有所区别,尤其是损害的类型以及成因。山海关这边沿海,粉化和泛碱这两个类型是我们之前没有遇到过的。数据集建立以后,识别的精度也比之前的研究有所提升。
论文标题框架:
1. Introduction
1.1. Research Background
1.2. Literature Review
1.3. Problem Statement and Objectives
2. The Great Wall and Influential Climate Factors
2.1 Climate Characteristics of Qinhuangdao
2.2. Analysis of the Architectural Features of the Shanhaiguan Great Wall
2.3. Damage Types and Cause Analysis of the Shanhaiguan Great Wall
3. Methods
3.1. Research Process
3.2. Sample Processing
3.3. Model Training
4. Discussion: Automatically Identifying and Analyzing the Results
4.1. Model Testing
4.2. Model Application
4.3 Main limitations and wider applicability
5. Conclusions
Data availability statement
Author contributions
Publisher’s note
References
Appendix A: Machine learning environment
引用格式:Li Q, Zheng L, Chen Y, Yan L, Li Y and Zhao J (2023), Non-destructive testing research on the surface damage faced by the Shanhaiguan Great Wall based on machine learning. Front. Earth Sci. 11:1225585.doi: 10.3389/feart.2023.1225585
线上阅读地址:https://www.frontiersin.org/articles/10.3389/feart.2023.1225585/full
3. 论文插图
![[JCR]Great Wall on Machine Learning [JCR]Great Wall on Machine Learning](https://scitip.com/wp-content/uploads/2023/09/image-9-939x1024.png)
Figure 1. World Heritage scope of the Great Wall of Shanhaiguan (Image Source: https://whc.unesco.org/en/list/438/maps/).
![[JCR]Great Wall on Machine Learning [JCR]Great Wall on Machine Learning](https://scitip.com/wp-content/uploads/2023/09/image-10-878x1024.png)
Figure 2. The climate of Qinhuangdao. (Image Source: drawn by the author).
![[JCR]Great Wall on Machine Learning [JCR]Great Wall on Machine Learning](https://scitip.com/wp-content/uploads/2023/09/image-11.png)
Figure 3. Structural schematic of the Plain Great Wall of Shanhaiguan. (Image Source: drawn by the author).
![[JCR]Great Wall on Machine Learning [JCR]Great Wall on Machine Learning](https://scitip.com/wp-content/uploads/2023/09/image-12-1024x372.png)
Figure 4. Damage types and label names of the gray bricks of the Shanhaiguan Great Wall. (Image Source: the picture was taken by the author, and the text was drawn and added by the author. The shooting date was April 24, 2023).
![[JCR]Great Wall on Machine Learning [JCR]Great Wall on Machine Learning](https://scitip.com/wp-content/uploads/2023/09/image-13-1024x434.png)
Figure 5. The influences of climate factors on damage types. (Image Source: drawn by the author).
![[JCR]Great Wall on Machine Learning [JCR]Great Wall on Machine Learning](https://scitip.com/wp-content/uploads/2023/09/image-14-1024x253.png)
Figure 6. Research process with seven steps. (Image Source: drawn by the author).
![[JCR]Great Wall on Machine Learning [JCR]Great Wall on Machine Learning](https://scitip.com/wp-content/uploads/2023/09/image-15-1024x355.png)
Figure 7. Photos of gray bricks possessed by the Shanhaiguan Great Wall. (Image Source: photographed by the author).
![[JCR]Great Wall on Machine Learning [JCR]Great Wall on Machine Learning](https://scitip.com/wp-content/uploads/2023/09/image-16-1024x964.png)
Figure 8. YOLOv4 network framework used in this study. (Image Source: drawn by the author).
![[JCR]Great Wall on Machine Learning [JCR]Great Wall on Machine Learning](https://scitip.com/wp-content/uploads/2023/09/image-17-1024x612.png)
Figure 9. The LOSS values of the model training process. (Image Source: drawn by the author).
![[JCR]Great Wall on Machine Learning [JCR]Great Wall on Machine Learning](https://scitip.com/wp-content/uploads/2023/09/image-18-878x1024.png)
Figure 10. The outcomes of various weight file tests. The test samples are selected at random from the 361 training set samples (labeled A to F), and different weight files are loaded into the model to generate the results. (Image Source: drawn by the author).
![[JCR]Great Wall on Machine Learning [JCR]Great Wall on Machine Learning](https://scitip.com/wp-content/uploads/2023/09/image-19-1024x720.png)
Figure 11. The effect of the layer’s feature extraction process on the detection obtained for the test image. (Image Source: drawn by the author).
![[JCR]Great Wall on Machine Learning [JCR]Great Wall on Machine Learning](https://scitip.com/wp-content/uploads/2023/09/image-20-1024x534.png)
Figure 12. The effect of the layer feature extraction process on on-site photo detection. (Image Source: drawn by the author).
![[JCR]Great Wall on Machine Learning [JCR]Great Wall on Machine Learning](https://scitip.com/wp-content/uploads/2023/09/image-21-1024x385.png)
Figure 13. The detection effects produced for various photographs of construction sites. (Image Source: drawn by the author).
![[JCR]Great Wall on Machine Learning [JCR]Great Wall on Machine Learning](https://scitip.com/wp-content/uploads/2023/09/image-22-1024x597.png)
Figure 14. Manual model validation. (Image Source: drawn by the author).
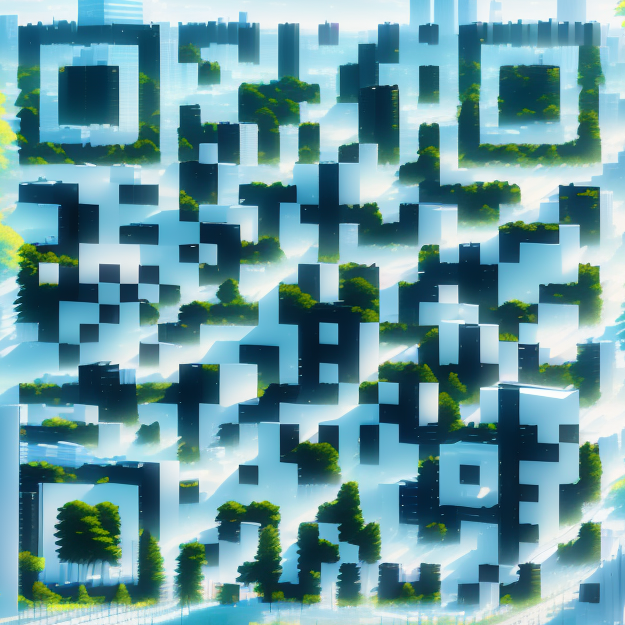